Nikolaj Thams
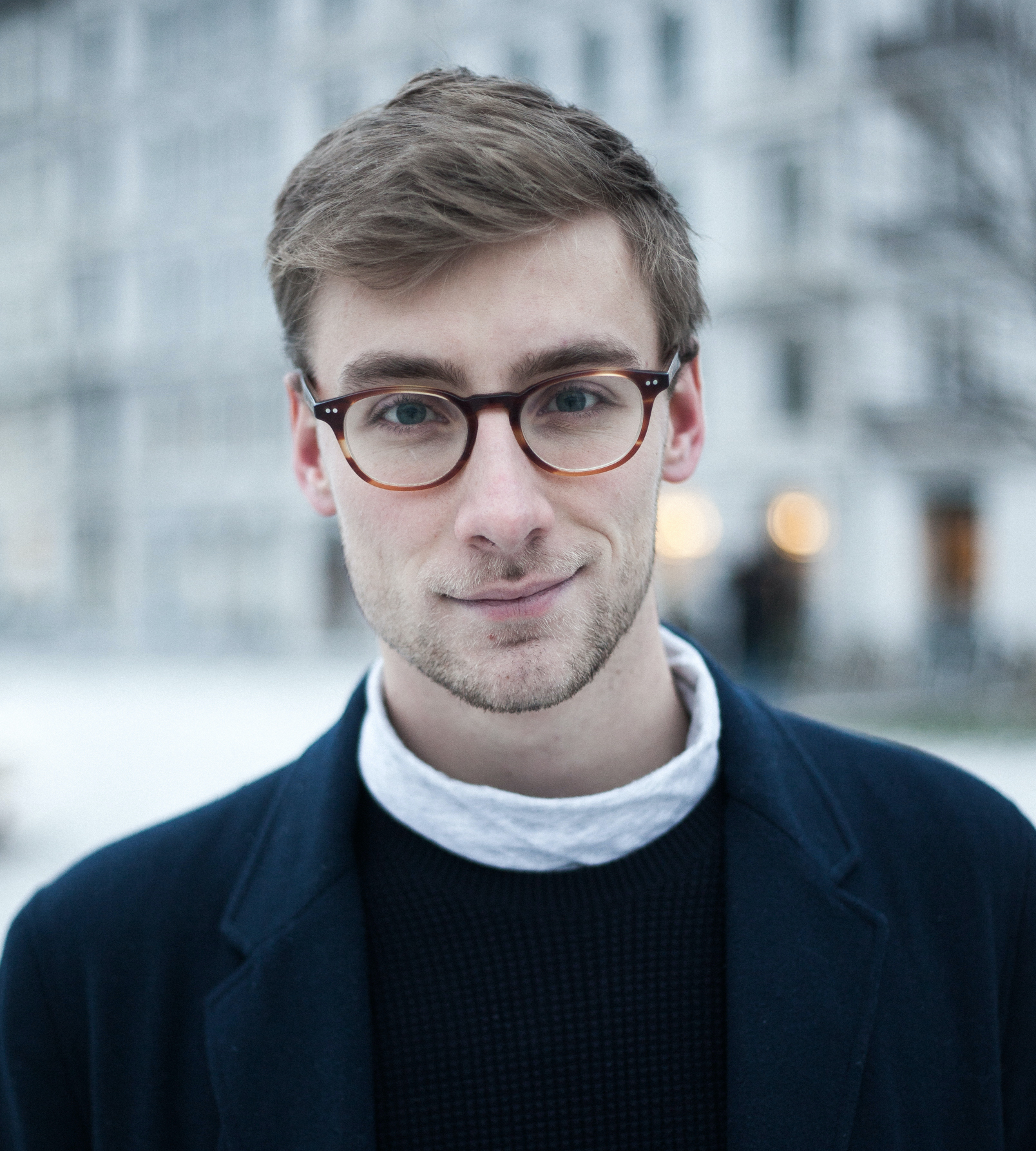
I am a Quantitative Researcher at G-Research in London. Previously, I was a PhD student of Statistics at the Copenhagen Causality Lab with Prof. Jonas Peters.
I have two bachelor degrees in Mathematics and Mathematical Economics and a master degree in Statistics, all from the University of Copenhagen. During my studies, I have spent semesters at University of Aarhus, University of Hong Kong, ETH Zürich and the Massachusetts Institute of Technology.
My research interests are at the intersection of Machine Learning and Mathematical Statistics, including causal inference, graphical models, optimization and dynamical systems. Feel free to reach out!
news
Sep 20, 2024 | Almost exactly five years after our first meeting about instrumental variable biases in timeseries, Identifying Causal Effects using Instrumental Time Series: Nuisance IV and Correcting for the Past, with Rikke Søndergaard, Sebastian Weichwald and Jonas Peters was accepted at JMLR today! |
---|---|
Feb 24, 2024 | Family‐based preventive intervention for children of parents with severe mental illness: A randomized clinical trial, with Anne Dorothee Müller, Ida Gjøde and others is now published in JCPP Advances. I very much enjoyed the collaboration! |
Nov 26, 2023 | Our paper Local Independence Testing in Point Processes, with Niels Richard Hansen, was accepted at IEEE Transactions on Neural Networks and Learning Systems. |
Nov 27, 2022 | Two papers accepted: “Evaluating Robustness to Dataset Shift via Parametric Robustness Sets” (w/ M. Oberst and D. Sontag) was accepted at NeurIPS 2022 and “Statistical Testing under Distributional Shifts” (w/ S. Saenkyongam, N. Pfister and J. Peters) at the JRSS-B ![]() |
Jun 02, 2022 | My research visit at the ClinicalML lab is coming to an end, and I have had a great time here! I’m very grateful to David Sontag for hosting me! Together with Michael Oberst, I have been working on an interesting project: Evaluating Robustness to Dataset Shift via Parametric Robustness Sets. We argue that considering bounded parametric shifts allow for interpreting the worst-case performance of a model, and we propose a second-order approximation of the loss under shift, which has a particularly pretty interpretation, when the shift occurs in an exponential family model. [PDF] [Code] |
May 16, 2022 | Great news: Our paper on Invariant Ancestry Search, with Phillip Mogensen and Jonas Peters was accepted at ICML 2022 ![]() |
Mar 14, 2022 | New paper Identifying Causal Effects using Instrumental Time Series: Nuisance IV and Correcting for the Past online now! In this paper, we provide a structured treatment of instrumental variable (IV) methods in time series data, by showing that valid IV models can be identified by representing the time series as a finite graph. We emphasize the need for adjusting for past states of the time series, to get valid instruments, and develop Nuisance IV, a modification of IV estimators. [PDF] [Code] |
Feb 01, 2022 | In our new paper Invariant Ancestry Search, we learn causal ancestors by using heterogeneity in data. We test sets for minimally invariance, and show that the union of minimaly invariant sets is ancestral. Our method retains level guarantees also when dimensionality is too large to exhaustively search all sets, and we apply our method in a genetic dataset with 6000 genes. [PDF][CODE] |
Oct 26, 2021 | In our new work, Local Independence Testing in Point Processes, we develop methods for learning causal structure in complex event data, such as firing of neurons in the brain. [PDF][CODE] |
Jun 04, 2021 | When dealing with policy data from several environments, some predictors may be great in some environments, but not in others. In our new paper Invariant Policy Learning, we show that learning invariant sets of predictors allows us to learn policies that generalize well to new environments. [PDF] |
May 24, 2021 | What do you do if you observe data from a distribution Q but want to test a hypothesis about a different, but related, distribution P ? In our recent paper, Statistical Testing under Distributional Shifts, we show how to test hypotheses in P by applying a test in a auxiliary dataset drawn by weighted resampling. [PDF] |
Mar 03, 2021 | Predictive models are great, but models that predict well when environments change are even better! Our new work, Regularizing towards Causal Invariance, on generalization from proxy variables, with Michael Oberst, Jonas Peters and David Sontag, is now on arXiv. [PDF] |
Mar 03, 2020 | Are p-values better better for detecting causal links than raw regression coefficients? Not always, we demonstrate in the paper Causal Structure Learning from Time Series, which is now on arXiv. [PDF] |
Dec 14, 2019 | In October, we participated in the Causality4Climate NeurIPS challenge, and it was just announced that we won! Thanks to the organizers for hosting, and for letting us present our winning solution at NeurIPS! [Code solutions] [Slides] [University news] |
Dec 02, 2019 | I’m going to be the webmaster and workflow chair for the UAI 2020 conference. Please shoot me any suggestions you may have for the webpage. |